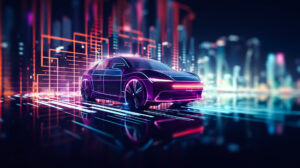
Understanding customer needs is a crucial aspect of the automotive industry. In fact, the industry has been quick to adopt these technologies in recent years, owing to its ability to deliver valuable insights into customer preferences and behavior.
According to a report by Capgemini, the share of automotive companies deploying AI at scale grew marginally to 10% compared to 7% in 2017. The number of selective AI implementations (at multiple sites in an organisation, but not at enterprise scale) has not moved significantly. It stood at 24% in January 2019, versus 27% in 2017. That being said, the global market for AI in the automotive industry is expected to grow at a CAGR of 55% from 2023 to 2032, with the market size expected to reach $600 billion by 2032.
The adoption of AI in the automotive industry has been driven by the need to improve safety, reduce costs, and enhance customer experience. AI has been used to develop advanced driver assistance systems (ADAS) that can detect and respond to potential hazards on the road. AI has also been used to optimise supply chain management, improve manufacturing processes, and enhance the overall quality of vehicles.
A Merit expert says, “The growth of AI in the automotive industry is expected to continue in the coming years, with the development of autonomous vehicles and the increasing demand for connected cars. In fact, it’s evident that the use of AI in the automotive industry is set to revolutionise the way we travel and interact with our vehicles in the future.”
Understanding Customer Needs and Improving the Driving Experience
There are many ways in which AI and text analytics technologies are being used in the automotive industry. Let’s look at what they are;
- Analysing customer feedback: They’re being used to analyse customer feedback, social media posts, and other data sources to identify customer preferences and pain points. This information is then used to improve vehicle design, features, and services. For example, Ford used AI/ML to analyse customer feedback on the F-150 pickup truck and identified that customers wanted more storage space.
- Predictive maintenance: These tools are being used to predict when a vehicle will need maintenance. This can help prevent breakdowns and reduce repair costs. For example, AI/ML tools can be used to analyse data from sensors in the vehicle to predict when a part is likely to fail.
- Autonomous driving: AI/ML tools are being used to develop autonomous driving technology. This technology is being used to improve safety, reduce traffic congestion, and increase fuel efficiency. For example, Tesla is using AI/ML to detect obstacles and navigate the road. Though the system has been involved in several accidents, the company is continuing to develop the technology.
- Supply chain management: These tools are being used to optimise supply chain management. This can help reduce costs and improve efficiency. For example, AI/ML tools can be used to predict demand for parts and optimise inventory levels.
Challenges in Adoption of AI &Text Analytics Technologies
The automotive industry has been adopting AI and text analytics to improve the efficiency of their operations and provide better services to their customers. However, this adoption has not been without its challenges.
Schema mapping of varying data sets
One of the biggest challenges is the complexity of the technology.
AI and text analytics require a significant amount of data to be effective, and automotive companies need to ensure that they have the necessary infrastructure in place to handle this data. For example, Tesla collects data from its vehicles to improve its self-driving technology. The company has a fleet of over 1 million vehicles, which generates a massive amount of data.
Lack of AI expertise
Another challenge is the lack of skilled talent in the field of AI and data science. Automotive companies need to invest in training their employees and hiring new talent to ensure that they have the necessary expertise to implement these technologies. For instance, General Motors has invested in training its employees in data science and AI to develop its autonomous vehicle technology.
Data privacy and security
The industry collects a significant amount of data from vehicles, and this data needs to be protected from cyber threats and other security risks. For example, in 2021, a hacker was able to access the source code of Tesla’s Autopilot system and demanded a ransom.
Regulatory compliance in the use of data in AI
As AI and text analytics become more prevalent in the automotive industry, companies need to ensure that they are complying with all relevant regulations and standards. For example, the European Union has introduced the General Data Protection Regulation (GDPR), which regulates the collection and use of personal data.
Despite the challenges, the automotive industry is expected to continue to invest in AI and text analytics in the coming years. The use of AI and machine learning in the automotive industry is expected to grow by 39% between 2021 and 2026, with the global market for AI in the automotive industry expected to reach $10.8 billion by 2026.
The use of AI and text analytics in the automotive industry is expected to improve manufacturing processes, increase supply chain efficiency, and make driving safer, more comfortable, and more entertaining. With the increasing demand for connected cars, AI and text analytics will play a crucial role in providing better services to customers and improving the efficiency of operations.
Merit’s Expertise in Data Aggregation & Harvesting for the Global Automotive Sector
Merit Data and Technology excels in aggregating and harvesting automotive data using AI, ML, and human expertise. Our capabilities include:
- Crafting end-to-end data pipelines and scalable data warehouses
- Designing compliant governance solutions for seamless integration
- Utilising high-volume, high-velocity data tools for nuanced insights
- Extracting retail product attributes and audience data
- Aggregating industry-specific data points for informed decision-making
Trusted by leading automotive brands, Merit drives innovation and efficiency by delivering refined, actionable insights.
Key Takeaways
AI’s Impact on Customer-Centric Innovation:
- Customer Feedback Analysis: AI and text analytics are employed to dissect customer feedback, social media posts, and data, shaping vehicle design, features, and services based on identified preferences and pain points.
- Enhanced Driving Experience: Predictive maintenance ensures timely vehicle upkeep, preventing breakdowns, and reducing repair costs, while autonomous driving technologies driven by AI aim to revolutionise safety, traffic congestion, and fuel efficiency.
Challenges Faced by the Automotive Industry:
- Technological Complexity: The adoption of AI and text analytics is hindered by the complex nature of these technologies, demanding robust infrastructure capable of handling massive datasets generated by a fleet of vehicles.
- Talent Shortage: A lack of skilled talent in AI and data science necessitates investments in training existing staff and recruiting new expertise to successfully implement these technologies.
- Data Privacy and Security: The industry grapples with safeguarding vast amounts of collected vehicle data from potential cyber threats and security risks, exemplified by instances like the 2021 Tesla Autopilot system breach.
- Regulatory Compliance: As AI becomes more prevalent, companies must navigate and comply with evolving regulations, such as the GDPR in the European Union, which governs the collection and use of personal data.
Future Prospects and Industry Growth:
- Continued Investment: Despite challenges, the automotive industry is expected to persist in investing in AI and text analytics, with a projected 39% growth in the use of AI and machine learning between 2021 and 2026.
- Global Market Projection: The global market for AI in the automotive industry is anticipated to reach $10.8 billion by 2026, highlighting the industry’s commitment to leveraging these technologies for improved manufacturing, supply chain efficiency, and enhanced driving experiences.
- Connected Cars and Efficiency Gains: With increasing demand for connected cars, AI and text analytics are set to play a pivotal role in delivering superior services to customers, fostering operational efficiency, and shaping the future of the automotive landscape.
Related Case Studies
-
01 /
A Hybrid Solution for Automotive Data Processing at Scale
Automotive products needed millions of price points and specification details to be tracked for a large range of vehicles.
-
02 /
Automotive Data Aggregation Using Cutting Edge Tech Tools
An award-winning automotive client whose product allows the valuation of vehicles anywhere in the world and tracks millions of price points and specification details across a large range of vehicles.