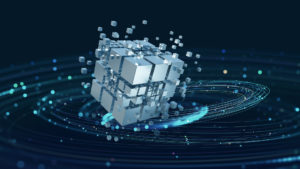
Data is an organisation’s most valuable asset – even if it doesn’t own it. So long as it has access, and the data is current and accurate, it can use it to generate insight and make better business decisions.
The amount of data a business can access increases year on year.
Where once it might only have had its own metrics to call on, today’s data-centric enterprises frequently supplement their own assets with second- and third-party data to produce a more nuanced and insightful result.
This level of sophistication is achieved at Merit with AI driven data transformation tools that are augmented by experienced data scientists. These tools help inform and drive intelligence in a broad range of fields as diverse as marketing, news media extraction and monitoring evolving international regulatory requirements.
Traditionally, data would have been stored on site, and looked after by a dedicated systems engineering team. However, the quantities of data that are now relied upon by enterprises increasingly makes this impractical – and neither is it cost-effective, when stood beside cloud alternatives.
The Benefits of Cloud Data Warehouse Solutions
By outsourcing to a cloud data warehouse provider, businesses can scale as required, reconfigure with ease, and rely on bespoke, specialist support to maintain and optimize the back end. Of these, the ability to scale is perhaps the most important, with storage and compute functions effectively uncoupled, allowing for an increase in one without requiring a simultaneous investment in the other.
“Having a data warehouse in the cloud improves the overall value of the data warehouse,” say Sherry Tiao and Keith Laker at Oracle. “It means that business intelligence and other applications can deliver faster, smarter insights to the business since the availability, scalability and performance are better.”
As a data solutions provider, Merit uses cloud data warehouses as part of its delivery for clients. This allows it to scale, ensuring that it always has the necessary resources to deal with even the largest data sets. While processes can be hosted entirely in the cloud, hybrid environments, incorporating on-premises processing, can also be implemented where appropriate, depending on a client or job’s specific requirements.
Data Warehouse configuration
Depending on the warehouse’s configuration, enterprises may also benefit from improved availability through duplication of sites and physical links, and more effective security by obscuring the location of the servers running their processes.
Restrictions of Data Warehousing Solutions
Compromises must still be made, though. While it’s possible to shop around, and occasionally to mix-and-match technologies, enterprises that turn to cloud data warehouse providers may not have total control over every layer of the technology stack, and their choice may be restricted by geographic considerations, where legislation limits the transfer and processing of data beyond specified borders.
Choosing a Cloud Data Warehouse Provider
From experience, we know that care must be taken when choosing a provider, for although one of the benefits of cloud over on-premises hosting is the ability to migrate to an alternative provider with minimal downtime, it is still preferable to avoid this if possible.
Understanding Your Requirements
It is important that the enterprise understands its own technology requirements before talking to potential providers. Discussions are likely to encompass data and query types, and data formats. The hardware and software stack must be capable of hosting the necessary architecture and allow for simultaneous use of multiple data sources. Where data must be archived for future reference, or to comply with legal requirements, cold storage options, at lower cost, with the same provider are highly beneficial, as they significantly reduce complexity, and the chargeable workload involved in migrating old data from one warehouse to another. Providers may offer different grades of cold storage, such as Google’s Nearline, Coldline and Archive options for data required annually, quarterly, or monthly, respectively.
Supporting Infrastructure
Unless it is to replace existing infrastructure entirely, any cloud data warehouse must integrate with the tech that already underpins the enterprise in a secure and manageable manner. As Rackspace explains, “Most cloud-based data warehouses include support for virtual private networks with connectivity to on-premises networks utilizing industry-standard IPsec VPNs. Most organizations would need devoted in-house teams to manage this level of security infrastructure. Even with an in-house team, in most cases, organizations could not match the level of sophistication and ease of applying the security controls in a cloud environment.”
Considering the Cost of Data Warehouse Solutions
As with any purchasing decision, cost will naturally play a part. However, this should not be a primary consideration, since additional spend, compared to an existing on-premises operation, may be possible to write down against better insight.
“There’s no guarantee that you will realise any cost savings at all,” says Teresa Wingfield at Actian. “But let me suggest, however humbly, that this may be the wrong question. Even if you could save money—and you could—cost reduction shouldn’t be driving your cloud data warehouse strategy. The strategic benefits that arise from moving to a cloud data warehouse should be driving that strategy, and for this reason your organization can gain valuable operational and strategic advantages even if you don’t reduce your costs.”
Data warehouses and data virtualisation
Much of this improved insight will be facilitated by the ability of cloud data warehouses to gather multiple data sets to produce more meaningful, contextualized results.
Through data virtualisation, these diverse data sets can be presented as though they were physically located in a single location, even though they may be drawn from a diverse range of sources – and locations. This greatly simplifies the process of querying the data, allowing business decision makers to self-serve rather than relying on a centralised IT support team to parse queries on their behalf. This accelerates the speed of business, allowing them to test and iterate within their decision-making process, while all the time working on the most recent data set available.
Use cases for data virtualisation
Because the various data sources are available at a single location, they can be used to provide summary output for further analysis through data aggregation.
Once an aggregation query has been established, with a defined reporting period, refreshing to the output at regular intervals will always present the enterprise with a live summary of the current situation. Thus, it may use data aggregation to calculate average revenue per customer, which may be subject to seasonal fluctuations. Or, the same data sources may be aggregated to demonstrate the impact of weather changes on footfall, using a combination of third-party (weather) and first-party (visitor) metrics.
Data virtualisation is particularly well suited to analytical activities of this kind as opposed to transactional database use in which users would instead be adding, amending, or deleting data.
Although the enterprise might not be directly manipulating the data it’s working with, analytical activities frequently require them to perform operations on complete data sets, rather than individual rows. Thus, the scalability of cloud greatly facilitates operations of this kind, since it allows the organisation to spin up additional computing resources when required, to be retired again when needs are reduced.
Data Aggregation and the Semantic Layer
Aggregation is just one model of cloud data warehouse use. When data is virtualised, its value is multiplied many times over. The ability to combine and contrast it with companion data sets in simultaneous or serial queries gives the results greater context and meaning.
Standardising Data with a Semantic Layer
The process of mining this kind of information can be greatly simplified, for end users, by deploying a semantic layer. This introduces applies a degree of standardisation across the data sets, allowing columns and tables, which may have bespoke names in different databases, to be referenced using a common set of agreed terms that make more sense to the end user.
A semantic layer, explains Kyvos, “maps complex data into familiar business terms so that users across the enterprise can access the same source of truth, with full confidence in its integrity. The idea is to get all the definitions and business logic in one place and then manage and change them centrally. The basic purpose of the semantic layer is to make data more useful to the business and simplify querying for the users.”
Thus, should one database be so designed that footfall is accounted for in a ‘visitors’ variable, and in another by a ‘customers’ variable, the semantic layer could standardize on one or the other, or replace both with ‘clients’, ‘check-ins’ or an alternative term of their choice.
How Data Scientists Deal with Variables in the Semantic Layer
So long as the semantic layer is maintained to accommodate new data sources as they become available, the data scientists – or business managers – working with the data inside their preferred BI tool, will thus be freed of any obligation to learn and conform with multiple incompatible structures when they query multiple databases simultaneously. So long as they know the agreed term for each variable used within their organisation, they can deploy it in their queries, and rely on the semantic layer to translate it into the equivalent term for each data source and, in each instance, return the relevant metric.
Cloud data warehouses and insight
Cloud data warehouses are the power behind the data-centric enterprise. By facilitating efficient data virtualisation, they make a wider and more flexible range of resources accessible to users across the organisation. These users, in turn, are working with common BI tools and, courtesy of a semantic layer, employing the terms they already understand to self-serve their own queries.
The result is more meaningful insight, and the ability for stakeholders throughout the organization to model outcomes and, on that basis, drive change, in ways that would have been difficult – if not impossible – using offline tools.
Related Case Studies
-
01 /
Automotive Data Aggregation Using Cutting Edge Tech Tools
An award-winning automotive client whose product allows the valuation of vehicles anywhere in the world and tracks millions of price points and specification details across a large range of vehicles.
-
02 /
Formularies Data Aggregation Using Machine Learning
A leading provider of data, insight and intelligence across the UK healthcare community owns a range of brands that caters to the pharmaceutical sector and healthcare professionals in the UK.