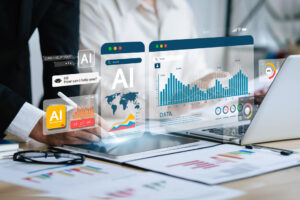
Big data has quietly infiltrated every corner of business, shaping decisions and strategies worldwide. By 2025, a staggering 463 exabytes of data will be generated daily—equivalent to around 212,765,957 DVDs. This data surge isn’t noise; it’s the lifeblood of organisations, driving an average 8% increase in profits for companies that embrace data solutions.
But, does Big Data by itself make sense?
Big data is the raw material gathered from various sources, but it makes sense when there is context, interpretation and decision-making from the data. This is where AI (artificial intelligence) comes into play. Whether it’s analysing sales figures, patient records, or market trends, AI, firstly, helps us understand data within its context. Secondly, by extracting and interpreting meaning from data, AI bridges the gap between data and actionable insights. Lastly, AI empowers decision-makers. From optimising supply chains to personalising marketing campaigns, data-driven decisions rely on AI’s ability to process information and recommend actions.
8 Ways AI has Revolutionised Big Data
Like we said earlier, firstly, AI algorithms have enabled the conversion of big data into actionable insights, empowering organisations to make informed decisions, optimise processes, and predict future trends. These algorithms have been able to enhance big data’s processing capabilities, facilitating the identification of complex connections, anomalies, and correlations that might otherwise remain unnoticed.
Additionally, AI has enabled the real-time analysis of massive data sets, delegating tasks such as pattern identification and learning to computer-based systems. This agility in analysing large volumes of data has enhanced decision-making processes by providing timely and relevant information.
Moreover, AI applied to big data has proven to eliminate analysis paralysis by swiftly providing clear and actionable directions from large data sets, thus avoiding inaccurate interpretations. With the increasing adoption of cloud computing and IoT sensors, the use cases for big data and AI have become more prevalent.
Furthermore, AI algorithms have automated data processing tasks, including data cleaning, transformation, and feature engineering, thereby reducing manual effort and accelerating the entire data pipeline.
AI-driven recommendation engines have leveraged big data to analyse user behavior and preferences, offering personalised content, product recommendations, and targeted marketing.
Predictive analytics models, such as regression, decision trees, and neural networks, have leveraged historical data to forecast trends, customer behavior, and market dynamics, informing strategic decisions.
Natural Language Processing (NLP) techniques powered by AI have been able to process unstructured text data, enabling applications such as sentiment analysis, chatbots, and language translation, extracting valuable insights from textual data.
Lastly, AI algorithms have been able to successfully identify anomalies or outliers in large datasets, enhancing data quality and security by detecting fraud, network intrusions, or equipment failures.
Shell plc, HSBC, BP, Rio Tinto, Lloyds Banking Group – each of these organisations have combined AI and big data to drive various business decisions. Shell plc, for example, uses AI for oil exploration, supply chain optimisation, and predictive maintenance. HSBC uses it for fraud detection, risk management and customer service. BP uses it for reservoir modeling, safety monitoring, and emission reduction, and so on.
The future of AI & Big Data
A Merit expert says, “In the coming years, AI’s impact on big data will continue to evolve, emphasising practicality, accessibility, and ethical considerations.” As businesses move beyond the initial hype surrounding Artificial Intelligence (AI), we can see a noticeable shift towards a more refined understanding of its capabilities. This evolution will bring about a recalibration of expectations, leading to a focus on practical applications rather than lofty promises. Moreover, there’s a growing recognition of the importance of responsible AI practices, with ethical considerations taking center stage. Ensuring that AI deployments adhere to ethical standards will become crucial in maintaining trust and avoiding potential pitfalls.
Another notable trend we can expect is the rise of multimodal AI systems capable of processing various data types, including text, images, and audio. These models can enhance understanding and decision-making by gathering insights from diverse information sources. Simultaneously, there will be a shift towards smaller language models, which are proving to be powerful alternatives to their larger counterparts. Open-source advancements, driven by community fine-tuning, are outperforming proprietary models, thus fostering accessibility and democratisation of AI.
However, challenges will come, such as GPU shortages driven by the escalating demand for AI infrastructure. To address this, companies need to explore cost-effective solutions, including optimising cloud usage and adopting hybrid setups. Additionally, techniques like quantisation and pruning will gain traction for model optimisation, while customised local models tailored to specific tasks will be increasingly favored.
When it comes to virtual agents and chatbots, AI-powered systems will evolve to handle more complex interactions, driven by improved natural language understanding and context-awareness. Nevertheless, as AI continues its march into various domains, regulatory frameworks around AI deployment and data privacy are inevitable. Companies will be expected to navigate these regulations while also addressing ethical concerns and biases inherent in AI systems.
Another emerging challenge is the prevalence of “shadow AI,” referring to unsanctioned AI deployments within organisations. To manage this, clear corporate policies will be expected to emerge, governing AI adoption and ensuring proper governance throughout the organisation’s AI initiatives.
Merit’s Expertise in Data Aggregation & Harvesting Using AI/ML Tools
Merit’s proprietary AI/ML tools and data collection platforms meticulously gather information from thousands of diverse sources to generate valuable datasets. These datasets undergo meticulous augmentation and enrichment by our skilled data engineers to ensure accuracy, consistency, and structure. Our data solutions cater to a wide array of industries, including healthcare, retail, finance, and construction, allowing us to effectively meet the unique requirements of clients across various sectors.
Our suite of data services covers various areas: Marketing Data expands audience reach using compliant, ethical data; Retail Data provides fast access to large e-commerce datasets with unmatched scalability; Industry Data Intelligence offers tailored business insights for a competitive edge; News Media Monitoring delivers curated news for actionable insights; Compliance Data tracks global sources for regulatory updates; and Document Data streamlines web document collection and data extraction for efficient processing.
Key Takeaways
- Big Data’s Ubiquitous Influence: Big data has become indispensable in shaping business decisions and strategies worldwide, with a staggering amount of data generated daily.
- The Role of AI in Unleashing Big Data’s Potential: While big data provides raw material, AI brings context, interpretation, and actionable insights to the table, enabling informed decision-making across industries.
- The Evolution of AI and Big Data: The convergence of AI and big data has revolutionised data analytics, enabling organisations to derive value from vast datasets through automation, real-time analysis, and predictive modeling.
- Practical Applications and Ethical Considerations: As businesses mature in their understanding of AI, there’s a shift towards practical applications and a heightened focus on responsible AI practices, emphasising ethical considerations and regulatory compliance.
- Emerging Trends: The future of AI and big data will witness the rise of multimodal AI systems, smaller language models, and open-source advancements. However, challenges such as GPU shortages and shadow AI deployments within organisations will need to be addressed.
- Integration in Business Operations: Leading organisations like Shell plc, HSBC, BP, and Rio Tinto have successfully integrated AI and big data into various aspects of their operations, driving efficiency, innovation, and strategic decision-making.
- Continued Evolution and Governance: Moving forward, the evolution of AI and big data will emphasise practicality, accessibility, and ethical considerations. Clear corporate policies and regulatory frameworks will be essential to govern AI adoption and ensure ethical AI practices across industries.
Related Case Studies
-
01 /
AI Driven Fashion Product Image Processing at Scale
Learn how a global consumer and design trends forecasting authority collects fashion data daily and transforms it to provide meaningful insight into breaking and long-term trends.
-
02 /
Bespoke Data Engineering Solution for High Volume Salesforce Data Migration
A global market leader in credit risk and ratings needed a data engineering solution for Salesforce data migration.