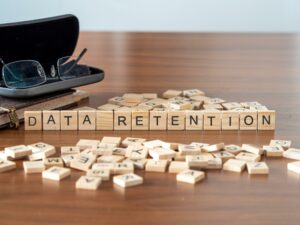
Cloud platforms like AWS and Azure offer cost-effective storage options for data that is rarely accessed.
Services like Azure Archive Blob Storage and AWS Glacier storage service are designed to cater to storage needs like backup, archiving, disaster recovery, and certain big data analytics requirements. While such storage options do exist, it is critical for any organisation to optimise for infrastructure and have a well-documented data storage strategy in place.
Specifically, technology leaders and CIOs must craft a data retention strategy for different types of data sets and have a plan in place to delete older data. This will not only reduce the cost of storage but also play a key role in shaping the overall cloud management strategy. Needless to say, it also helps with better data integrity, data governance, data security, and overall data management capabilities.
In this blog, our focus will be on highlighting the need for a data retention game plan, as more and more business applications move to the cloud.
What is data retention?
Data retention refers to the practice of storing and managing data for a pre-decided time duration. When a company is planning its cloud strategy, data retention is one of the most important factors to consider.
Data needs to be retained, stored, and accessible for various reasons; In some cases, legacy data may be used for BI and analytics purposes. In certain other cases, regulatory compliance will demand that certain types of records and documents be stored for several years. There is also the case of retaining data for disaster recovery or business continuity planning.
Additionally, it is not only about data storage but also about defining policies around privacy, governance, and access to this data. Who has permission to access certain data sets? How are privacy regulations being enforced? All these are questions that need to be answered.
Data Retention Policy 101: Need for a documented strategy
Today, technology leaders who are responsible for cloud strategy and migration, are putting in place a Data Retention Policy document that is drafted in consultation with various stakeholders.
The document typically includes the following sections:
- What types of data need to be retained? For what duration?
- What is the best storage service to be used to retain this data?
- If at all this data needs to be accessed, how can that be done?
- When can we confidently delete this data?
- How can we ensure privacy, security, and governance policies are met?
- Who holds approval rights on this data to make decisions around when this data can be deleted?
- How can policy or privacy violations be handled?
CIOs also believe that this data retention policy must be a dynamic document that gets improved with time. It can sometimes be tempting to set aggressive policies around how long certain records or documents are stored, but you never know when a particular legacy record may be needed. It is, therefore, critical to put in place a data retention strategy that considers various scenarios.
Data Retention for Analytics: The need for Data Classification
From an analytics perspective, data classification is a critical requirement to figure out whether a data set can be deleted or not. Data classification is nothing but the process of organising data by category and usage.
Let us say, for instance, there are legacy data sets from several years back that is stored in an ERP like Oracle EBS. The latest operational data probably resides in a cloud-based solution like Oracle Fusion, but the legacy data may come in handy while building analytical models for Business Intelligence (BI). In such cases, it is important to retain transactional data from several years back, even though there is no compliance or regulatory requirement.
A Merit expert says, “In such scenarios where long-term retention becomes the norm, it may make sense to implement a data warehouse solution like Amazon Redshift, and ensure that compute and storage resources are split optimally and strategically. It may be ideal to implement a Lakehouse architecture using Amazon S3 with Redshift.”
Depending on the data retention strategy, Amazon S3 lifecycle rules will come into play and different storage options can be used for different time durations. For instance, S3 Glacier will be used for long-term retention of say 5–12 years, and S3 Standard and S3-IA can be used for data that needs to remain accessible for less than 5 years.
There are also equivalent storage options available in both Azure and GCP ecosystems.
Merit’s Expertise in Cloud Migration and Data Retention Efforts
Merit works with a broad range of clients and industry sectors, designing and building bespoke applications and data platforms combining software engineering, AI/ML, and data analytics.
We migrate legacy systems with re-architecture and by refactoring them to contemporary technologies on modern cloud ecosystems. Our software engineers build resilient and scalable solutions with cloud services ranging from simple internal software systems to large-scale enterprise applications.
We can also help your enterprise strategise and plan out a data retention strategy, keeping in mind compliance, regulatory, analytics, and business requirements.
To know more, visit: https://www.meritdata-tech.com/service/code/digital-engineering-solutions/
Related Case Studies
-
01 /
A Digital Engineering Solution for High Volume Automotive Data Extraction
Automotive products required help to track millions of price points and specification details for a large range of vehicles.
-
02 /
Advanced ETL Solutions for Accurate Analytics and Business Insights
This solutions enhanced source-target mapping with ETL while reducing cost by 20% in a single data warehouse environment