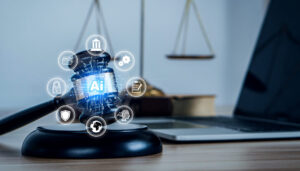
The past few years have witnessed a significant global surge in AI adoption, with companies across various industries actively integrating AI into their operations. Notably, in 2022 53% of IT companies accelerated their AI adoption, a substantial increase from the 43% reported previously. The AI technologies market itself is slated to touch USD 1.8 trillion by 2030, from USD 200 billion in 2023.
Amidst this rapid expansion and adoption, the ethical implications of AI have come to the forefront. As AI permeates deeper into society, concerns regarding ethics have intensified. Issues such as algorithmic bias, data privacy, and the societal impact of automation have propelled discussions on ethical AI implementation to the forefront of global discourse.
In this blog, we discuss why ethics in AI really matters, the current approach to AI ethics, challenges and limitations to these approaches, and how ethical considerations can be put into practice.
Why Does Ethics in AI Matter?
Given this rapid expansion of AI, ethical considerations have become increasingly critical. Here’s why:
- Bias and Fairness: AI algorithms learn from historical data, which may contain biases. If these biases are not addressed, AI systems can perpetuate discrimination, reinforce stereotypes, and lead to unfair outcomes. Ethical AI development involves actively identifying and mitigating bias to ensure fairness.
- Transparency: AI models can be complex and difficult to interpret. Transparency is essential to build trust with users and stakeholders. Ethical AI developers strive to make their models explainable, allowing users to understand how decisions are made.
- Privacy: AI systems often process personal data. Protecting user privacy is essential. Ethical AI development involves robust privacy practices, data anonymisation, and informed consent.
- Accountability: When AI systems make decisions, who is responsible? Ethical considerations emphasise accountability. Developers should be accountable for the behavior of their AI models and address any unintended consequences.
- Safety: AI systems can have real-world impact, from autonomous vehicles to medical diagnoses. Ensuring safety is critical. Ethical AI development includes rigorous testing, risk assessment, and fail-safes.
- Long-Term Impact: AI technologies shape our future. Ethical considerations extend beyond immediate use cases. Developers must think about long-term consequences, societal impact, and sustainability.
Current Approaches to Ethics in AI
In this section, we delve into various approaches that guide the responsible creation and deployment of AI systems. From foundational principles to interdisciplinary collaboration, these strategies shape the ethical landscape of AI technology.
1. Principles-Oriented Approaches
These approaches focus on defining ethical principles that guide AI development and deployment. Some common principles include fairness, transparency, accountability, and privacy. By adhering to these principles, AI practitioners aim to create systems that treat all individuals fairly and avoid bias.
2. Process-Oriented Approaches
Process-oriented approaches emphasise the integration of ethics into AI development processes. This involves creating frameworks, guidelines, and methodologies that ensure ethical considerations are part of every stage of AI system design, from data collection to deployment. By following robust processes, organisations can proactively address ethical challenges.
3. Ethical Consciousness and Individual Responsibility
Beyond formal frameworks, ethical consciousness plays a crucial role. AI practitioners need to be aware of the impact their work has on society. They should actively consider the consequences of their decisions, engage in ongoing learning about ethical issues, and take responsibility for the systems they create. This individual awareness contributes to a more ethically conscious AI community.
4. Value Alignment Approaches
Value alignment focuses on ensuring that AI systems align with human values and goals. Researchers explore methods to make AI understand and respect human preferences, cultural norms, and ethical standards. Techniques like inverse reinforcement learning and preference modeling fall under this category.
5. Contextual Ethics
Contextual ethics recognises that ethical considerations can vary based on the specific context in which AI operates. For example, an autonomous vehicle’s ethical decisions may differ depending on road conditions, passengers, and pedestrians. Researchers work on context-aware AI systems that adapt their behavior accordingly.
6. Interdisciplinary Collaboration
Ethical AI benefits from collaboration across disciplines. Computer scientists, ethicists, policymakers, and domain experts collaborate to address complex challenges. By combining technical expertise with ethical insights, we can create more robust and responsible AI systems.
Challenges & Critiques in AI Ethics
A Merit expert says, “One of the first things to consider when it comes to challenges, is that existing approaches to AI ethics face significant challenges and critiques. Despite efforts to create fair and unbiased AI systems, inherent biases persist due to biased training data and algorithmic design, resulting in discriminatory outcomes.”
Additionally, many AI models, particularly deep learning ones, lack transparency, making it difficult to understand their decision-making processes. This opacity raises concerns about accountability and responsibility—determining who should be held accountable for AI decisions is complex and remains unresolved.
Privacy concerns also loom large as AI systems often collect and process vast amounts of personal data, raising questions about how to balance the benefits of AI with the protection of privacy rights. Recent scholarship has critiqued current ethical practices in AI, identifying common issues such as “ethical washing,” where organisations adopt ethical guidelines for PR purposes without genuine commitment, and the lack of diversity in AI development teams, which can perpetuate biases.
Moreover, many ethical frameworks prioritise short-term concerns over long-term consequences, potentially overlooking broader societal impacts. Critics argue that existing regulations are inadequate to address the ethical challenges posed by AI, highlighting the need for more robust and comprehensive regulatory frameworks to ensure ethical AI development and deployment.
Innovative Approaches: Narrative & Technology Ethics
To overcome these challenges and critiques, we can explore alternative approaches:
Narrative ethics, for one, offers a compelling approach to AI ethics, emphasising storytelling and empathy. Through narratives, stakeholders can gain a deeper understanding of the impact of AI on individuals and society. Instead of discussing AI ethics in abstract terms, developers can share personal stories related to their work, such as a machine learning engineer narrating their discovery and resolution of bias issues in a recommendation system. Similarly, an AI researcher could share their experience designing an explainable AI model and its impact on user trust. These narratives humanise the ethical challenges of AI development, fostering empathy among stakeholders.
Technology ethics advocates for the integration of ethical considerations directly into the design and development of AI systems, rather than treating AI as a separate entity. This approach entails embedding ethical principles into the technology itself. For instance, privacy-enhancing technologies such as differential privacy mechanisms, federated learning, and secure multi-party computation prioritise privacy and fairness during AI development. These technologies are designed to protect individual privacy while allowing for effective data analysis and collaborative AI without compromising sensitive information.
In addition to privacy-enhancing technologies, ethical AI design patterns play a crucial role in promoting responsible AI development. Similar to design patterns in software engineering, ethical AI design patterns guide developers toward responsible choices. For example, “red teaming” involves simulating adversarial attacks to identify vulnerabilities, ensuring robustness and security in AI systems. “Human-in-the-loop” systems ensure human oversight in critical AI decisions, enhancing transparency and accountability. Furthermore, “model cards” provide standardised documentation on model behavior and limitations, promoting transparency and enabling stakeholders to make informed decisions about AI usage. These patterns collectively promote transparency, accountability, and fairness in AI design and development.
8 Ways Ethical Considerations Can Be Practically Applied in AI
Early involvement of ethicists and stakeholders is crucial for ensuring ethical AI development. By including ethicists and domain experts from the outset of AI projects, their insights can guide ethical decision-making processes. Furthermore, engaging stakeholders, including users, affected communities, and regulators, allows developers to understand their concerns and values, ensuring that AI systems align with societal expectations and ethical standards.
To operationalise ethical considerations, it’s essential to define specific guidelines and principles for each project, aligning them with broader ethical principles such as fairness, transparency, and privacy. These guidelines should be translated into actionable rules for AI development, facilitating the implementation of ethical practices throughout the project lifecycle.
Bias mitigation and fairness are paramount in ethical AI development. This involves auditing training data for biases, monitoring model performance across different demographic groups, and using fairness-aware algorithms to mitigate bias during model training.
Transparency and explainability are essential for fostering trust in AI systems. Developers should choose interpretable models when possible, provide explanations for model predictions, and document model behavior and limitations to ensure transparency and accountability.
Privacy protection is another critical aspect of ethical AI development. Implementing privacy-enhancing techniques such as differential privacy, federated learning, and homomorphic encryption helps safeguard sensitive information. Minimising data collection and retaining only necessary information further enhances privacy protection.
Human oversight and accountability are integral to ethical AI deployment. Designing human-in-the-loop systems allows human reviewers to validate AI decisions and escalate complex cases to human experts. Establishing clear lines of responsibility for AI outcomes ensures accountability and mitigates risks.
Testing and validation processes should include checks for ethical violations, evaluating model behavior on edge cases and adversarial examples, and checking for discriminatory outcomes. Additionally, regular audits and updates of ethical guidelines are necessary to adapt to evolving societal norms and emerging risks.
Education and training initiatives are essential for equipping developers and data scientists with the necessary knowledge and skills to navigate ethical implications and apply best practices in AI development. Collaboration with the wider AI community through conferences, workshops, and forums facilitates knowledge sharing and collective learning, fostering a culture of ethical AI development across organisations and industries.
The Evolving Landscape of AI Ethics
As AI advances, ethical considerations evolve. With autonomous systems, determining accountability becomes complex, requiring legal frameworks to adapt. International collaboration is vital for establishing consistent ethical guidelines and regulations, preventing misuse. In critical domains like healthcare and finance, ensuring ethical AI practices is essential to prevent harm and bias. Human-AI collaboration will increase, necessitating ethical guidelines to support this partnership while safeguarding human rights. Efforts to reduce bias and enhance fairness in AI algorithms will persist. Demands for transparent AI systems will rise, with users expecting explanations for AI decisions. Balancing AI capabilities with privacy protection remains crucial, especially in decision-making areas like hiring and criminal justice.
Keeping all this in mind, the future of AI ethics lies in collaborative efforts, global standards, and responsible development.
Merit’s Expertise in Data Aggregation & Harvesting Using AI/ML Tools
Merit’s proprietary AI/ML tools and data collection platforms meticulously gather information from thousands of diverse sources to generate valuable datasets. These datasets undergo meticulous augmentation and enrichment by our skilled data engineers to ensure accuracy, consistency, and structure. Our data solutions cater to a wide array of industries, including healthcare, retail, finance, and construction, allowing us to effectively meet the unique requirements of clients across various sectors.
Our suite of data services covers various areas: Marketing Data expands audience reach using compliant, ethical data; Retail Data provides fast access to large e-commerce datasets with unmatched scalability; Industry Data Intelligence offers tailored business insights for a competitive edge; News Media Monitoring delivers curated news for actionable insights; Compliance Data tracks global sources for regulatory updates; and Document Data streamlines web document collection and data extraction for efficient processing.
Key Takeaways
- The surge in AI adoption globally underscores the critical importance of addressing ethical considerations in AI development.
- Ethical AI development involves addressing bias and fairness issues, ensuring transparency, protecting privacy, establishing accountability, prioritising safety, and considering long-term societal impacts.
- Various approaches, including principles-oriented, process-oriented, ethical consciousness, value alignment, contextual ethics, and interdisciplinary collaboration, guide responsible AI development.
- Challenges such as bias in algorithms, lack of transparency, privacy concerns, and inadequate regulation persist in AI ethics.
- Innovative approaches like narrative ethics and technology ethics offer new perspectives in addressing ethical challenges in AI development.
- Practical applications of ethical considerations include early involvement of ethicists and stakeholders, defining ethical guidelines, bias mitigation, transparency, privacy protection, human oversight, testing, education, and collaboration.
- The evolving landscape of AI ethics necessitates global collaboration, adherence to ethical standards, and responsible development practices to ensure AI benefits society while minimising harm.
Related Case Studies
-
01 /
Advanced ETL Solutions for Accurate Analytics and Business Insights
This solutions enhanced source-target mapping with ETL while reducing cost by 20% in a single data warehouse environment
-
02 /
AI Driven Fashion Product Image Processing at Scale
Learn how a global consumer and design trends forecasting authority collects fashion data daily and transforms it to provide meaningful insight into breaking and long-term trends.