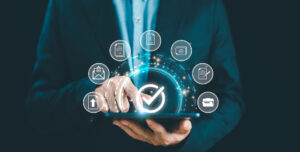
In today’s data-driven landscape, the quality of information is paramount. Organisations, businesses, and individuals increasingly rely on data for decision-making, strategic planning, and innovation. But why is data quality so vital?
High-quality data—free from errors, inconsistencies, and inaccuracies—forms the bedrock of reliable insights. It ensures accurate analysis and informed decision-making. Moreover, organisations seek actionable insights to stay competitive, optimise processes, and drive growth. Insights empower them to adapt swiftly to changing markets and customer needs.
Role of AI in Data Quality & Reliability
In recent years, Artificial Intelligence (AI) has played a pivotal role in enhancing data accuracy and reliability across various domains. Let’s explore the different ways in which AI has revolutionised data quality and reliability;
Accuracy Enhancement: AI-powered machine learning algorithms models excel in processing complex data by learning from historical patterns, identifying anomalies, and autonomously detecting inaccuracies within datasets. Additionally, AI facilitates efficient data cleansing through methods like scrubbing and deduplication, automatically pinpointing and rectifying errors, inconsistencies, and duplicate entries. This streamlined approach not only ensures data quality but also optimises the overall data management process, empowering organisations to rely on more reliable insights for informed decision-making and strategic planning.
Bias Mitigation: Ethical considerations in AI have led to a heightened focus on minimising biases inherent in data. By actively addressing biases during the training of AI models, we mitigate the risk of perpetuating unfair treatment towards specific groups or individuals. Moreover, ensuring diversity and representation in datasets is crucial. A diverse and representative dataset enables AI models to generalise effectively across various contexts and user demographics, thereby bolstering the reliability and fairness of AI-driven decisions and outcomes. These efforts are pivotal in fostering trust and accountability in the deployment of AI technologies across industries and applications.
Predictive Analytics: AI plays a critical role in enhancing system reliability through predictive capabilities. By predicting potential system failures, AI optimises maintenance schedules, leading to reduced downtime and prolonged operational longevity. Additionally, AI utilises predictive analytics to forecast emerging trends in data quality, preemptively identifying and mitigating issues before they affect insights. Real-time monitoring further enhances the reliability of outcomes, ensuring that organisations can rely on accurate and timely information for decision-making and strategic planning.
Challenges in AI Data Interpretation
AI algorithms designed for data cleansing bring powerful capabilities but often require structured data, posing a challenge since a substantial amount of real-world data remains unstructured. Initial data structuring and cleaning processes still heavily rely on human input to organise and prepare data effectively. However, AI’s potential for misinterpreting data or making incorrect assumptions necessitates human oversight to ensure accurate results throughout the cleaning process. Data quality issues, stemming from human error, system glitches, or integration problems, further underscore the need for robust AI tools capable of addressing missing values, duplicates, and outliers. Despite AI’s efficiency in expediting data cleaning tasks, human expertise remains indispensable in validating data quality and ensuring the accuracy and reliability of AI-driven solutions. This collaboration ensures that organisations can leverage both AI’s capabilities and human insights to achieve optimal data quality and informed decision-making.
What can we expect in the future?
AI-driven data cleansing tools are rapidly evolving, offering organisations automation, accuracy, and significant time savings. Looking ahead, future trends indicate a shift towards more autonomous AI systems in data cleansing. These advanced systems will independently detect and resolve data anomalies, streamlining the cleansing process with enhanced efficiency. Additionally, probabilistic computing is poised to play a pivotal role, enabling AI to make informed judgments based on uncertain data through sophisticated statistical methods, thereby improving overall data cleaning accuracy. Moreover, the integration of Large Language Models (LLMs), originally developed for natural language processing tasks, promises to revolutionise data cleansing workflows by providing scalable and efficient solutions to transform chaotic datasets into structured and usable information. Together, these advancements underscore AI’s transformative impact on enhancing data quality and operational effectiveness across diverse industries.
Merit’s Expertise in Data Aggregation & Harvesting Using AI/ML Tools
Merit’s proprietary AI/ML tools and data collection platforms meticulously gather information from thousands of diverse sources to generate valuable datasets. These datasets undergo meticulous augmentation and enrichment by our skilled data engineers to ensure accuracy, consistency, and structure. Our data solutions cater to a wide array of industries, including healthcare, retail, finance, and construction, allowing us to effectively meet the unique requirements of clients across various sectors.
Our suite of data services covers various areas: Marketing Data expands audience reach using compliant, ethical data; Retail Data provides fast access to large e-commerce datasets with unmatched scalability; Industry Data Intelligence offers tailored business insights for a competitive edge; News Media Monitoring delivers curated news for actionable insights; Compliance Data tracks global sources for regulatory updates; and Document Data streamlines web document collection and data extraction for efficient processing.
Key Takeaways
Importance of Data Quality: In today’s data-driven landscape, high-quality data is essential for reliable insights, accurate analysis, informed decision-making, and organisational agility in responding to market changes.
Role of AI in Data Quality and Reliability:
- Accuracy Enhancement: AI-driven machine learning algorithms excel in processing complex data, identifying anomalies, and automating data cleansing processes like scrubbing and deduplication.
- Bias Mitigation: AI addresses biases in data through ethical considerations and ensures diversity in datasets, enhancing the fairness and reliability of AI-driven decisions.
- Predictive Analytics: AI enhances system reliability by predicting failures, optimising maintenance schedules, and preemptively identifying data quality issues through predictive analytics.
Challenges in AI Data Interpretation: Despite AI’s capabilities in data cleansing, challenges such as the need for structured data and the potential for misinterpretation highlight the ongoing necessity of human oversight to ensure accurate results and validate data quality.
Future Trends in AI-driven Data Cleansing: The future of AI in data cleansing points towards more autonomous systems, probabilistic computing for uncertain data judgments, and integration of Large Language Models (LLMs) for scalable and efficient data transformation.
Related Case Studies
-
01 /
Automated Data Solution For Curating Accurate Regulatory Data At Scale
Learn how a leading regulatory intelligence provider is offering expert insights, analytics, e-Learning, events, advisory and consulting focusing on the payments and gambling industries
-
02 /
Enhanced Audience Data Accuracy for a High Marketing Campaign RoI
An international market leader in exhibitions within the learning, healthcare, technology and veterinary sectors.