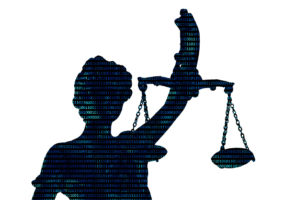
There are many instances in which it is no longer possible for human analysts to draw meaningful, actionable intelligence from data. There are simply too many metrics, arriving too quickly.
The only logical solution is to apply artificial intelligence – AI – informed by machine learning to each data set, to identify patterns, correlate, and project likely outcomes. It is perhaps not surprising, then, that this is one area technology is advancing at an unprecedented rate.
“According to a study done by OpenAI the amount of computing power used in AI training has doubled every 3.4 months. This is a massive, almost impossible acceleration that the standard progression is not used to,” writes Thomas David at Towards Data Science. “A concept used in computing is called Moore’s Law which estimates that computing power doubles every two years, The computing described above produces seven times more than Moore’s Law.”
The Use of AI in enterprises
McKinsey’s most recent State of AI report, published in December 2021, noted that “AI adoption is continuing its steady rise: 56 percent of all respondents report AI adoption in at least one function, up from 50 percent in 2020… The top three use cases are service-operations optimisation, AI-based enhancement of products, and contact-center automation, with the biggest percentage-point increase in the use of AI being in companies’ marketing-budget allocation and spending effectiveness.”
These use cases are unsurprising. AI doesn’t tire and is less likely to make mistakes than human operators when working with massive data sets, such as those generated by iterative product development loops, voice recognition, and response analysis, which are core, respectively, to each of the use lead cases.
“Automated insights and operations speed up processes and decrease the chances of user error,” says Shelby Hiter at Datamation. She includes further development of automated curation, smart factories, and more effective data mining among 2022’s top priorities for businesses deploying AI.
Hyper-automation through AI
AI has long been sold less as a way of replacing the human workforce, and more as an opportunity for organisations to deploy those expensive human assets on tasks to which they are best suited while reserving methodical, repetitive tasks for automation. It seems unlikely this will continue.
As explained by David De Cremer and Garry Kasparov in the Harvard Business Review, “machines have evolved to the point where they can now do what we might think of as complex cognitive work, such as math equations, recognising language and speech, and writing. Machines thus seem ready to replicate the work of our minds, and not just our bodies.
Merit’s Senior Service Delivery Manager, Mohamed Aslam says: “Given that major industries are well established across the globe, competition is at its highest. It’s important that services are rendered at the quickest turnaround along with the best solution and service rather than just focusing on shipping out commodities and products. Delivery alone no longer cuts it given that consumers are spoilt for choice. Hence, Hyper-Automation is critical for organisations to remain relevant.”
Is AI evolving to surpass human intelligence?
In the 21st century, AI is evolving to be superior to humans in many tasks, which means that we seem ready to outsource our intelligence to technology. With this latest trend, it seems like there’s nothing that can’t soon be automated, meaning that no job is safe from being offloaded to machine”.
It seems clear, then, that AI, in its current implementation, is merely a starting point, rather than our destination. We are approaching the point of hyper-automation, where businesses deploy a range of technologies and disciplines to automate as many processes as possible.
Gartner’s Fabrizio Biscotti described hyper-automation as having “shifted from an option to a condition of survival”. Gartner itself predicted that through 2024, “Technologies to automate content ingestion, such as signature verification tools, optical character recognition, document ingestion, conversational AI and natural language technology (NLT) will be in high demand… Gartner expects that by 2024, organisations will lower operational costs by 30% by combining hyper-automation technologies with redesigned operational processes.”
AI Wins with Processing, but Loses with Dependency
As it becomes adept at simulating human behaviour across a broader range of fields, the intelligence itself will feel increasingly authentic, until we reach the point where, for the end user, it will not only be impossible to tell whether they are benefiting from the labours of a human or a machine – but also irrelevant.
However, organisations must be mindful of the potential to implement an unfair transaction. AI relies on huge amounts of data, often gleaned from human subjects. This must be handled in a responsible manner.
Governance: A Key Principal of AI Ethics
“Governance, the fourth principal of AI ethics, is a necessary study to understand the outcomes of AI failures and push your organisation to apply high standards of risk management to your models,” explains DataRobot. “This is especially important in situations where life and death are at stake, such as hospital treatments and healthcare.”
But governance isn’t only beneficial to data subjects: without data governance, an organisation can’t be fully confident that the projections it’s developing and the actions it’s taking have a sound footing, since it can’t know whether the data on which they are based is reliable.
“The most important ingredient of machine learning is not power, because while higher processing power can train machines faster… it won’t change the quality of results,” writes AI researcher Brian Ka Chan. “Data is the bread and butter of machine learning and the worse the quality of the data you train with, the worse the result of the AI is. On the other hand, the better quality the data you bring in, the better the result it is.”
UK Laws Applicable to the use of AI
Although AI is not specifically mentioned in the EU’s GDPR, the legislation is relevant, since AI’s effective application frequently relies on the use of large quantities of personally identifiable or anonymised data. In particular, as Linklaters explains, “decisions taken by machine are directly subject to the GDPR, including the core requirement for fairness and accountability. In other words, the data subject can challenge the substantive decision made by the machine on the grounds that it is not fair and lawful”.
This is pertinent at a time when AI is increasingly used to, for example, pre-sift applicants for job vacancies. Research conducted by MIT Technology Review into the effectiveness of AI-driven candidate selection platforms found that, in some cases, the intelligence was tasked with discerning personality traits from intonation. Yet, Fred Oswald of Rice University told MIT Technology Review, intonation isn’t a reliable indicator of personality traits, and rather than using it for open-ended tests of this sort we should be focused on using it to gather structured data.
It could be argued, then, that responsibility lies with the AI’s human overseers to be analytical in their application of intelligence and machine learning, and not merely to apply it because they can.
Merit Group’s expertise in AI
At Merit Group, we work with some of the world’s leading B2B intelligence companies like Wilmington, Dow Jones, Glenigan, and Haymarket. Our data and engineering teams work closely with our clients to build data products and business intelligence tools. Our work directly impacts business growth by helping our clients to identify high-growth opportunities.
Our specific services include high-volume data collection, data transformation using AI and ML, web watching, BI, and customised application development.
The Merit team also brings to the table deep expertise in building real-time data streaming and data processing applications. Our data engineering team brings to fore specific expertise in a wide range of data tools including Airflow, Kafka, Python, PostgreSQL, MongoDB, Apache Spark, Snowflake, Tableau, Redshift, Athena, Looker, and BigQuery.
If you’d like to learn more about our service offerings, please contact us here: https://www.meritdata-tech.com/contact-us
Related Case Studies
-
01 /
Enhancing News Relevance Classification Using NLP
A leading global B2B sports intelligence company that delivers a competitive advantage to businesses in the sporting industry providing commercial strategies and business-critical data had a specific challenge.
-
02 /
High-Speed Machine Learning Image Processing and Attribute Extraction for Fashion Retail Trends
A world-leading authority on forecasting consumer and design trends had the challenge of collecting, aggregating and reporting on millions of fashion products spanning multiple categories and sub-categories within 24 hours of them being published online.